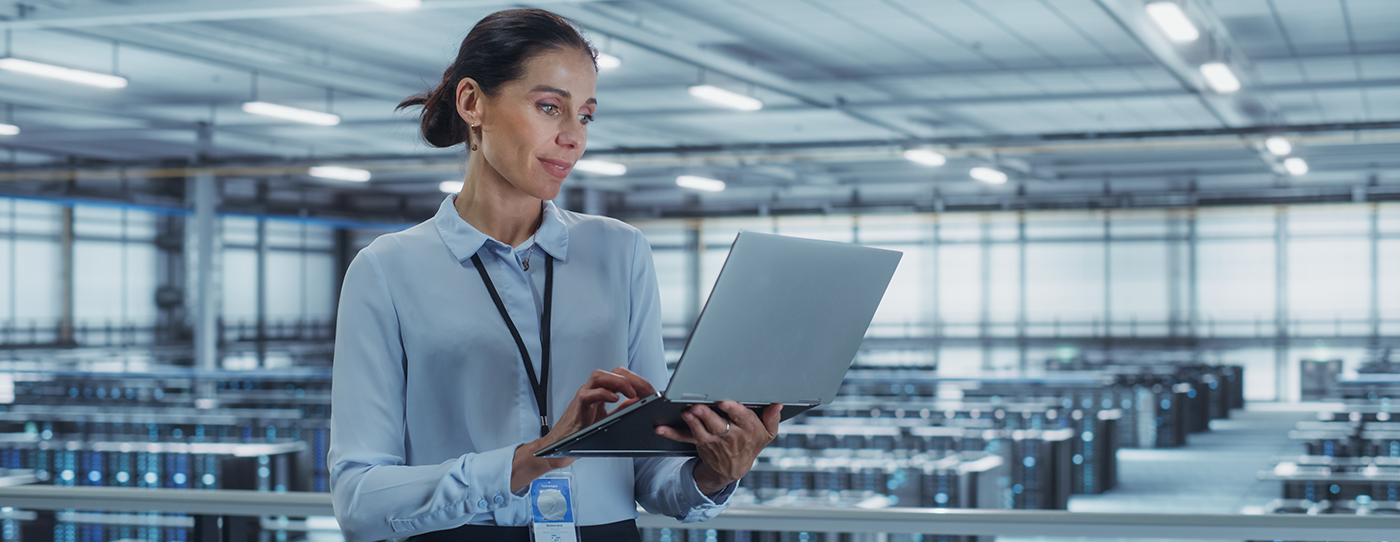
What is Data Mining? How Does it Contribute to Industrial Productivity?
In the modern digital era, the data produced by millions of individuals as part of their daily routines represents an extensive reservoir of valuable information. Our activities such as online engagements, social media updates, shopping patterns, and more collectively contribute to the generation of substantial data volumes. Nonetheless, the intricate and disorganized structure of this data presents a challenge when it comes to unveiling significant insights from it. This is precisely where the practice of data mining becomes crucial, as it serves the purpose of uncovering patterns, correlations, and meaningful revelations within sizable datasets, often denoted as big data.
Consequently, what exactly is data mining, what steps does it entail, and how does it play a role in enhancing industrial efficiency? Let's delve into exploring the solutions to these inquiries together.
What is data mining?
Data mining is a procedure that combines fields like statistics, artificial intelligence, and database management. Its objective is to unveil concealed and less apparent patterns and information within extensive datasets, often termed as big data. This procedure is usually executed through the utilization of data analysis techniques and algorithms, frequently recognized as machine learning. Data mining plays a role in forecasting forthcoming trends in the business realm, comprehending customer behaviors, molding marketing approaches, and attaining uncharted insights across diverse domains.
Data mining aids in forecasting upcoming patterns within the business sector, comprehending customer actions, molding marketing approaches, and acquiring novel insights in diverse fields.
What are the stages of data mining?
Data mining typically consists of the following stages:
Data Collection and Cleansing:The data mining process begins with the stage of data collection. The first phase entails the compilation of the datasets slated for analysis. These datasets commonly come from various origins and constitute significant reservoirs of extensive data. Following this, the accumulated data undergoes a process of purification and arrangement. During this phase, the elimination of inaccurate, incomplete, or incongruous data is pivotal in facilitating meaningful analysis. As an illustration of data collection, consider an e-commerce enterprise amassing data encompassing customer orders, product particulars, and preferences.
Data preprocessing:Once the dataset has undergone cleaning, the subsequent phase involves data preprocessing. During this stage, the data is readied to be suitable for analysis. Techniques encompassing feature extraction, feature selection, feature creation, and dimensionality reduction are applied to enhance the data's significance and utility. A feature represents a characteristic that can aid in differentiating one entity or variable from others, or in facilitating comparisons between variables. Illustrative instances of features encompass age, gender, and occupational category. In terms of feature values, the gender feature might encompass values like female and male, while the occupation group feature could involve values such as public, private, and self-employed. This phase results in the conversion of the data into a format that is more easily comprehensible and conducive to analysis.
Modeling:Modeling stands as a pivotal phase within the data mining process. During this stage, data sets undergo analysis through the utilization of data mining algorithms. This results in the revelation of patterns, relationships, and valuable insights embedded within the dataset. Diverse algorithms can be employed in this phase, often recognized as machine learning algorithms. A subset of these algorithms comprises:
- Classification and regression algorithms: Classification and regression algorithms serve the purpose of grouping data into distinct categories or making forecasts concerning existing conditions or forthcoming results using the data at hand. Instances of classification models encompass segregating customers according to their income tiers, while instances of regression models comprise estimating the potential shopping expenditure a customer might engage in. Fundamentally, classification models entail generating forecasts for categorical assignments, while regression models involve approximating continuous values.
- Clustering algorithms: Clustering algorithms work by arranging data into groups according to their inherent similarities. For example, clustering algorithms are favored when identifying customer segments.
- Association rules (Association analysis): Identifying the products or services that a customer is prone to buy during a shopping session or in successive shopping occasions holds significance. Association rules, which assist in recognizing buying patterns, find extensive use in data mining under the term 'market basket analysis,' primarily for marketing objectives.
Evaluation:Evaluating the performance of the created models holds significance. During this phase, the model's accuracy is validated, incorrect outcomes are rectified, and the model's effectiveness with real-world data is scrutinized.
Deployment:In the concluding phase, the achieved outcomes are incorporated into operational workflows or decision-making frameworks. Enterprises have the opportunity to formulate strategic choices, create novel products or services, and secure a competitive edge by leveraging these insights.
What are the contributions of data mining to industrial efficiency?
Upon incorporation into industrial operations, data mining has the potential to provide diverse benefits. Here are several domains in which data mining can enhance industrial efficiency:
Optimization of production processes
Data mining can aid in the enhancement of production processes through the utilization of data-driven insights. As an example, an automobile factory could scrutinize data obtained from its production lines to pinpoint stages that consume excessive time or experience diminished efficiency. This analysis could subsequently guide the restructuring of processes to elevate overall production efficiency.
Maintenance predictions and downtime reduction
Utilizing data mining, it becomes feasible to anticipate maintenance requirements for industrial machinery beforehand. To illustrate, a power plant could scrutinize data received from equipment sensors to predict potential malfunctions. This proactive approach can mitigate unscheduled downtime, curtail maintenance expenses, and enhance operational efficiency.
Customer demand forecasting
Utilizing data mining, it is possible to forecast customer demands. For example, a retail enterprise can examine historical sales data to predict which products will witness heightened demand during particular timeframes. As a result, superfluous inventory expenses can be diminished, all the while ensuring an appropriate product stock to fulfill demands.
Quality control and monitoring of errors
Data mining can be a favorable choice for implementing quality control within manufacturing lines. To illustrate, a food producer can analyze data that traces the production stages of items, enabling quality control measures. In the event of detecting an error or abnormal situation, the production line can be promptly halted to avert potential quality concerns.
Supply chain management
Utilizing data mining can yield positive outcomes in the realm of supply chain management. For instance, a logistics firm has the capacity to analyze variables like weather conditions and traffic, among others, to ascertain the optimal timing for shipments. This approach can lead to cost reduction alongside an improvement in customer satisfaction.
In summary, data mining involves the exploration of significant and applicable patterns within extensive datasets. Insights derived from the analysis of big data can play a role in enhancing the efficiency of processes.
At SOCAR Türkiye, we are consistently innovating methods to boost our industrial efficiency, leveraging our leading role in the sector. Our initiative, the 'HCU Diesel Flash Point Prediction and Optimization' project, has enabled us to forecast flash points in the diesel pool and diesel blend pool of the HCU unit using analytical models crafted through extensive big data analysis. Furthermore, we've incorporated an optimization model suggesting the infusion of heavy naphtha to attain the optimal flash point. The primary goal of this project is to secure financial advantages through loss prevention and amplified efficiency, stemming from streamlined management. This involves not only real-time calibration of flash points in the diesel pool but also the mitigation of quality-related losses. Guided by our commitment to energy, we're diligently working today to forge a more resilient tomorrow.